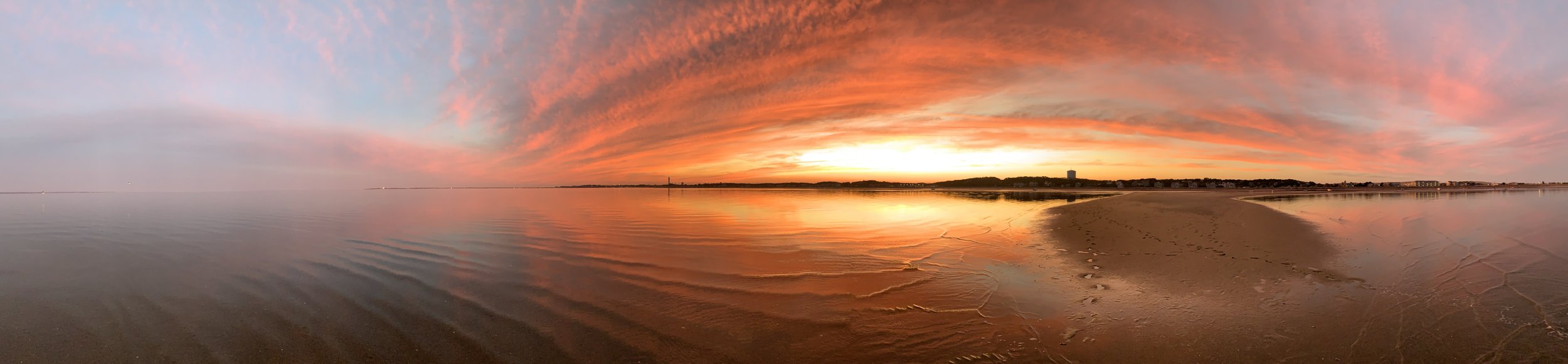

A Moment of Reckoning for Humanitarian and Public Sector
Leaders of these organizations now face a moment of reckoning and—in the chaos of that moment—an opportunity to craft the modern, technologically nimble institutions resilient enough to continue delivering the crucial services upon which their publics so desperately depend. They must use technology to drive out cost, with the core thesis being that to emerge stronger, they must become more modern organizations that use technology to reduce cost and improve services, rather than viewing technology as an ever-expanding cost center. Those that seize the opportunity may survive. Those who let it slip away will fail.

Applying Modern Ecosystem-Oriented Architecture in PubSec
We’ve covered the Principles of Ecosystem-Oriented Architecture (EOA) and Mapping your Cloud Ecosystem in previous articles. We’ll now make the concepts discussed in those previous articles more real in context of Public Sector organizations. To do so, let’s spend some time speaking less about technology and begin describing workloads that incorporate functions and scenarios upon which a typical agency might rely on its cloud ecosystem to perform.
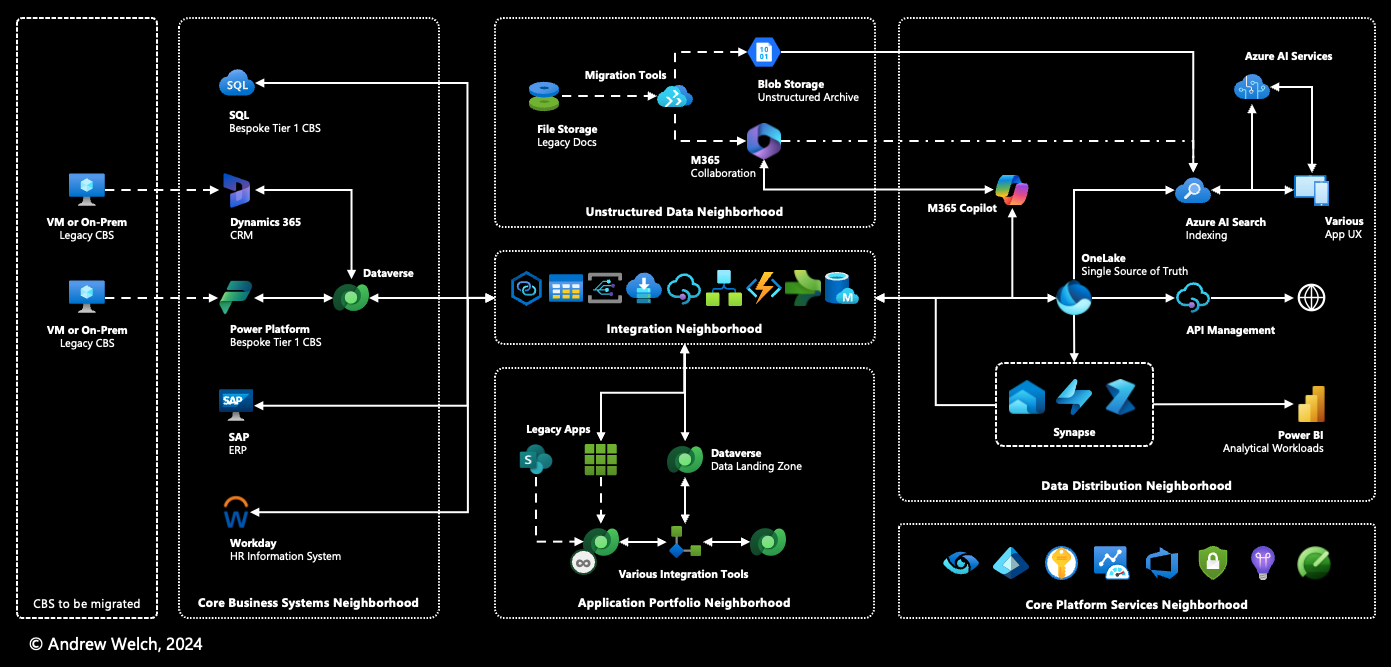
Mapping your Cloud Ecosystem: The Ecosystem Map
"Ecosystem Map" is both one of the 25 dimensions of the AI Strategy Framework and a foundational concept in ecosystem-oriented architecture (EOA), which makes this article doubly important reading for strategic thinkers on both fronts. The “map” metaphor is instructive here. It is used to distinguish an ecosystem map from the various forms of architectural diagrams, nearly all of which tend to include more technical minutiae than a typical ecosystem map. Whereas an architectural diagram provides specific parameters for specific technical solutions, an ecosystem map presents a higher-level, more visionary view of an organization’s cloud ecosystem. This analogy is fundamental to understanding and practicing EOA.

Principles of Ecosystem-Oriented Architecture
We were working with eight to ten years between major disruptions from the dawn of the consumer internet. But these “wave periods”, that is, the time between the crest of two waves, have shortened to three to five years since the rise of the public cloud. It makes sense: As the evolution of computing technology and capacity picks up steam, it similarly accelerates. Innovation begets innovation. Generative AI was only made possible by the incredible computing power and connectivity available in the cloud. Now, AI is further accelerating this pace of change, shortening the time we have available before new waves crash upon the shore. The grace period for organizations to get their act together and position themselves for the next wave is growing much shorter; the margin for error is much narrower.

Executive Vision and Actionable Roadmap for your AI Strategy
There’s an incredibly important transition in the broad information technology space that is often lost in the furor and excitement over generative AI. Simply “wanting AI” doesn’t cut it. So, the AI Strategy Framework begins with the Strategy and Vision pillar that sets forth five dimensions beginning with vision, extending to creating the actionable roadmap and architecture necessary to actualize that vision, and finally establishing the programmatic elements necessary to drive that vision to fruition. These dimensions help organizations formulate and take action on their big ideas.
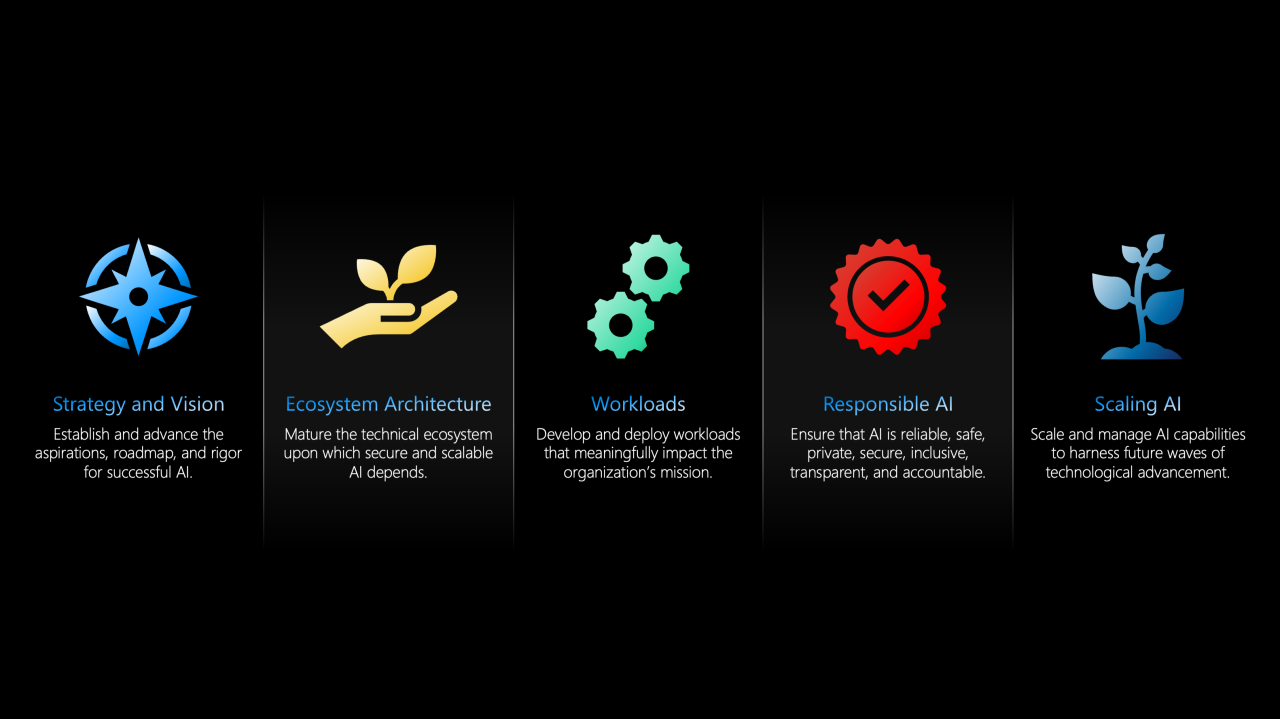
"AI Strategy Framework" guides your organization's journey in the Age of AI
We’ve learned a great deal about maturity and readiness for - and responsibility to the ethics of - AI over the past year, as well. It’s now time for a proper model through which organizations may realistically assess their readiness to adopt and scale artificial intelligence, and then identify specific areas to invest time, talent, and funding along their journey. This AI Strategy Framework guides organizations as they construct their AI strategy atop five pillars, each with five dimensions to be considered, matured, and regularly evaluated.
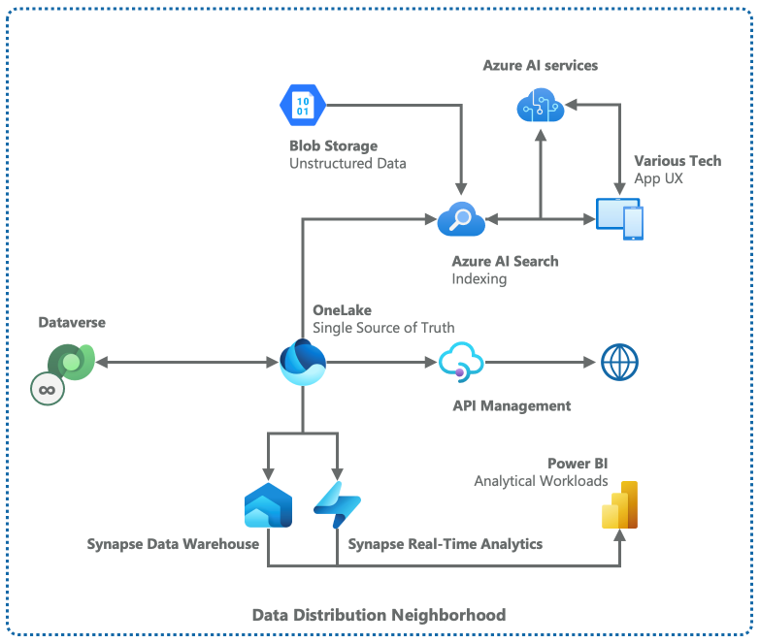
Data Distribution in Power Platform
When taking on the question of how Power Platform integrates with Azure data services, Point-to-Point, Data Consolidation, Master Data Node, and Data Distribution evolve a similar theme. Specifically, each focuses primarily on transactional data during any given users interaction with it. “Data Distribution” is different, focusing more on data distributed for analytics, enterprise search, integration with third-party or external sources via API, data science workloads, or training or augmenting a large language model (LLM). This blog overviews the Data Distribution pattern.
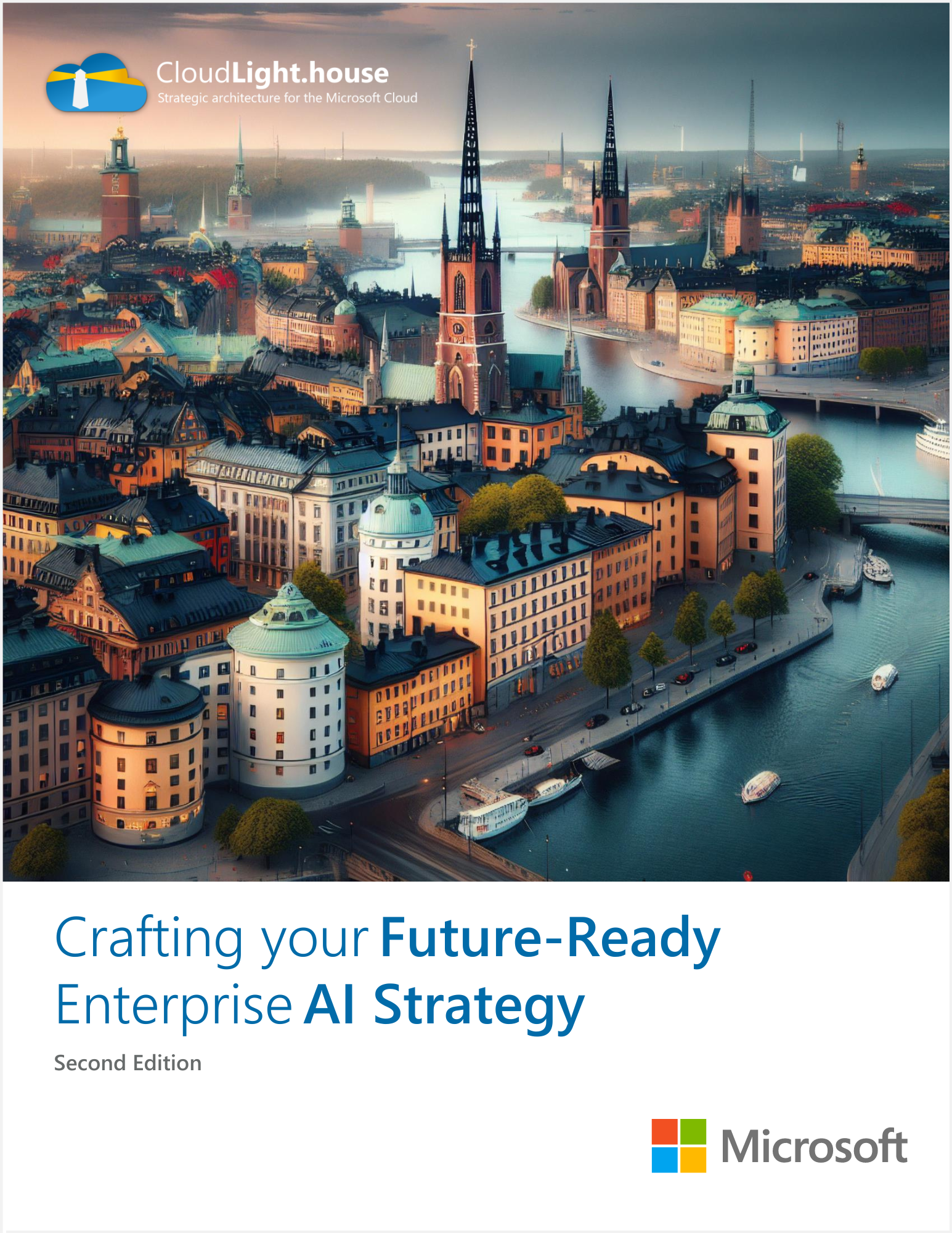
Whitepaper: “Crafting your Future-Ready Enterprise AI Strategy, e2”
It has become obvious how difficult so many organizations are finding it to actually craft and execute their AI strategy, in part because of the (often) decades they’ve spent kicking their proverbial data can down the road, in part because it turns out that enterprise-grade AI really does require the adoption of ecosystem-oriented architecture to truly scale, but largely because many organizations have no idea where to start. Many lack the wherewithal to really assess where they stand on day one, and to identify areas where they must mature to get to day 100 (and beyond).
We’ve learned a great deal about maturity and readiness for - and responsibility to the ethics of - AI over the past year, as well. It’s now time to broaden the thesis, so in this second edition we offer a model through which organizations may realistically assess their current maturity to adopt and scale artificial intelligence, and then identify specific areas to invest time, talent, and funding along their journey.
Whitepaper: “Ecosystem-Oriented Architecture in the Public Sector”
This whitepaper offers CIOs, enterprise architects, and other public sector technologists a comprehensive introduction underscoring the need to adopt ecosystem-oriented architecture (EOA) to build scalable, resilient, and flexible cloud ecosystems that can absorb successive waves of technological change.
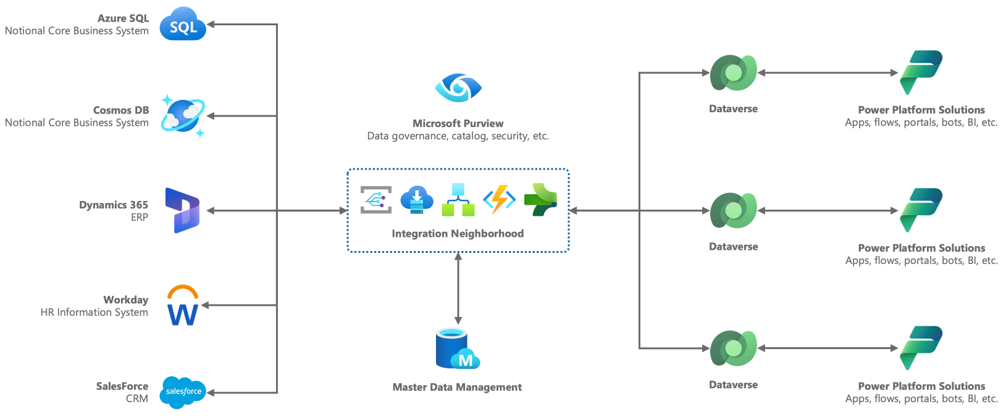
Five strategies to integrate Power Platform in your data platform architecture
When comparing architectural models for Power Platform, it’s important to avoid the instinct to choose just one. Instead, the goal is to explore various approaches that enable different scenarios for integrating Power Platform solutions with enterprise data. Each organization should strategically mix and match these approaches, considering factors like performance, flexibility, maintainability, and cost. This strategy allows for creating adaptable patterns within a cloud ecosystem where Power Platform plays a key role.

Whitepaper: “Scaling your Enterprise Cloud with Power Platform”
Power Platform has been woven into the fabric of enterprise IT for far longer than most people realize, and, as such, is often the backbone of mission-critical “Tier 1” workloads. This white paper is the technology leaders’ guide to strategic Power Platform in a modern cloud ecosystem, diving deep on some of the most important methods of scaling your entire enterprise cloud—data, AI, applications included—with Power Platform: Using Power Platform with your enterprise data, lowering your long-term costs, securing and governing your data to reduce risk, infusing AI into daily work, then scaling AI across your org, and scaling your cloud and other tech investments with specialized capabilities.
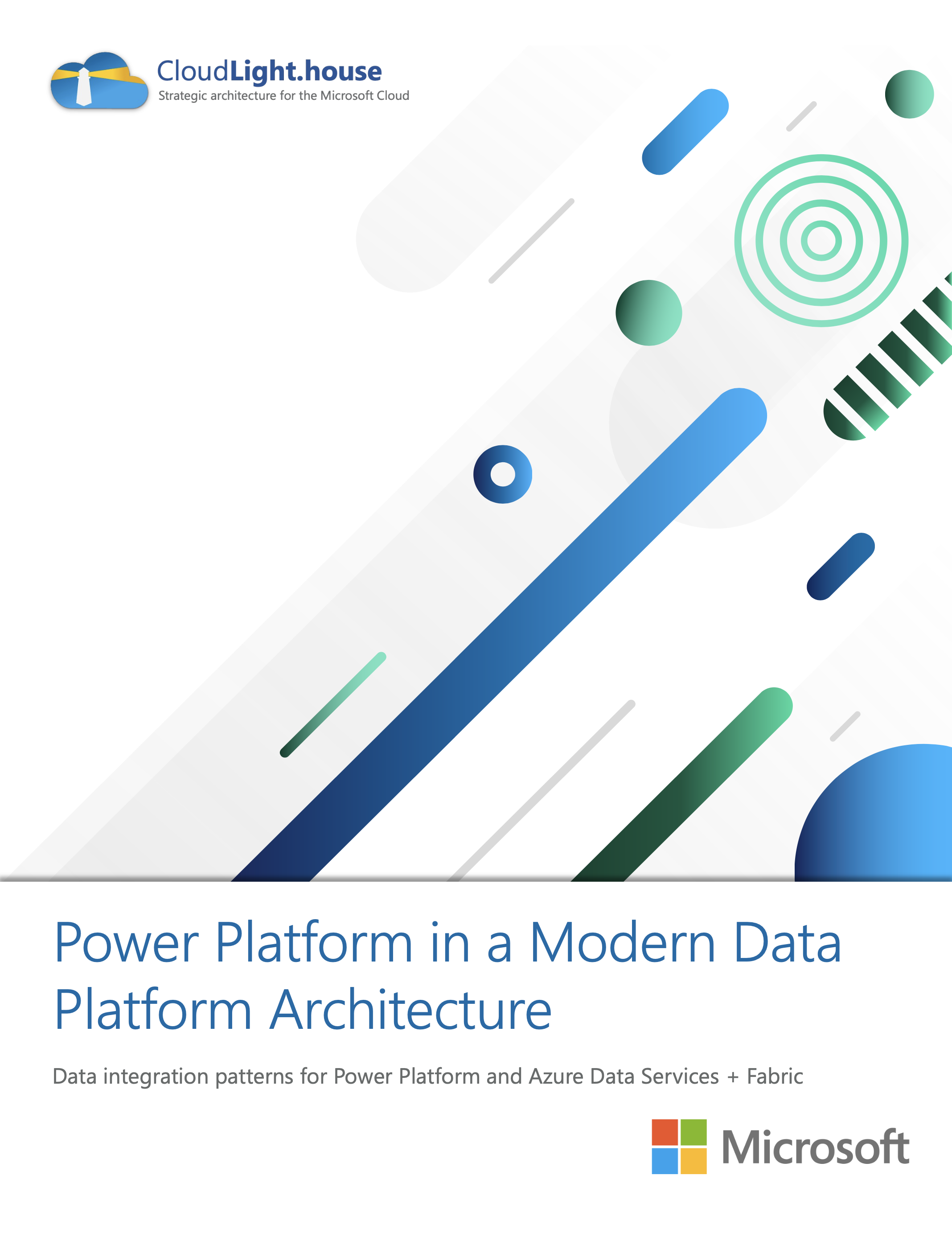
Whitepaper: “Power Platform in a Modern Data Platform Architecture”
For all the talk about Power Platform as a ‘’low-code’’ tool (and this is the last time I will use the word), for all the attention given to how supposedly easily it allows non-technical users to create simple apps, Power Platform’s greatest value lies not in the app, but in the data the app collects or serves back to its users. Power Platform isn’t an app phenomenon. It’s a data phenomenon. This white paper takes on the question of how Power Platform integrates with Azure data services including Microsoft Fabric, outlining five patterns that organizations ought to mix and match to extract Power Platform’s greatest value.
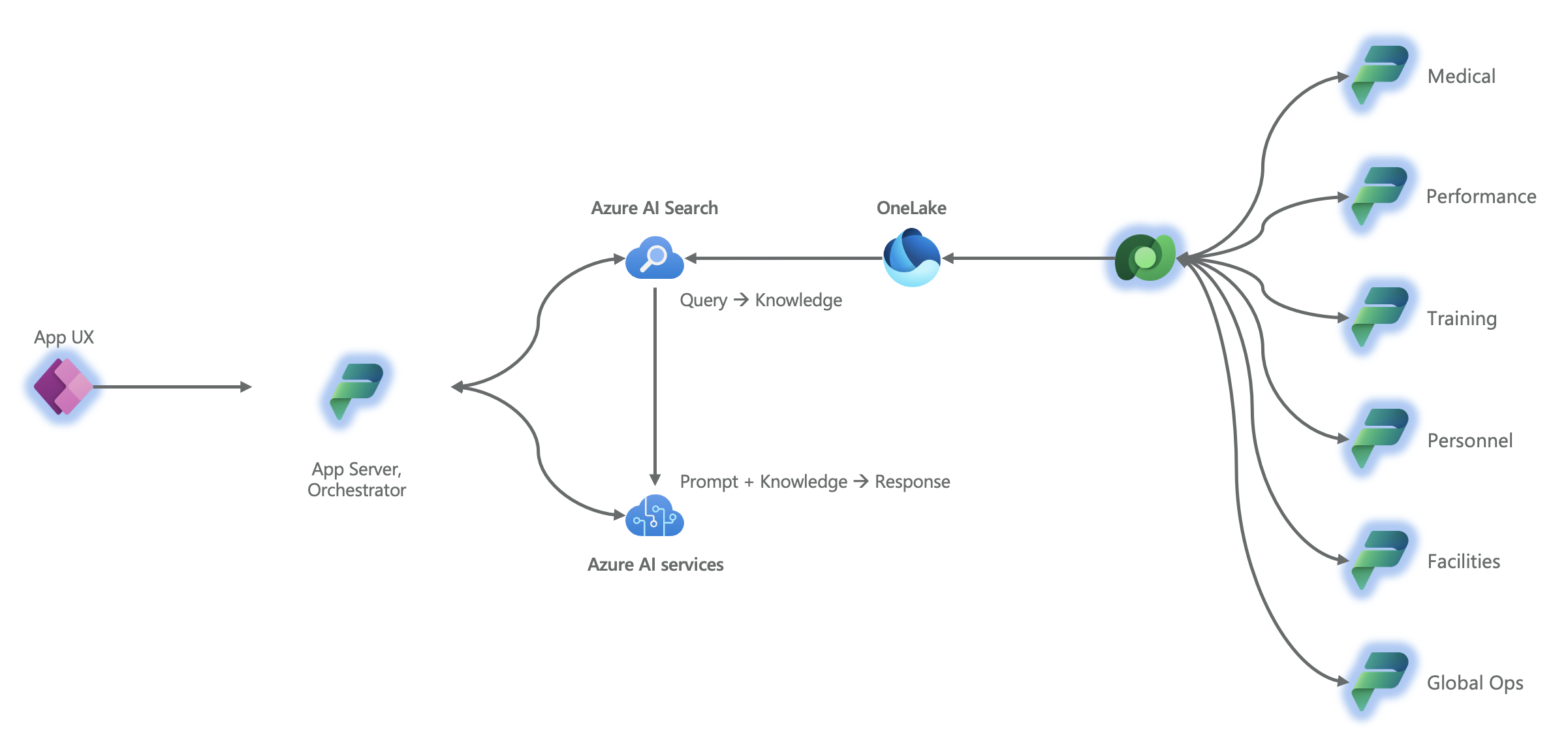
How Power Platform scales generative AI across an organization
Power Platform scales AI and the data platform by providing a composable means of both data collection and delivery of insights and AI capability back to the user. Meanwhile, the great, often unsung capability of Power Platform is not the “app”, rather the ability (via Dataverse) of data transacted in a Power Platform solution to hydrate downstream data distribution scenarios such as analytical workloads, enterprise search, and—you guessed it—whatever AI infused workload you dream up. Let’s explore this.

Whitepaper: “Crafting Your Future-Ready Enterprise AI Strategy”
It is yet unknown if artificial intelligence is more akin to a “great inventions” of the 19th and 20th centuries, or if it will ultimately represent another more incremental evolution of existing capabilities. The former—as seems more likely given the immense investments being made today—will present significant challenges to nearly every organization that, having become accustomed to incremental change, is suddenly faced with a “great inventions” caliber paradigm shift that AI seems to portend. This white paper explores foundational concepts for AI technology and your AI strategy, five pillars for your AI strategy—data consolidation, data readiness, incremental AI, differential AI, scaling AI—and trends that are likely to shape AI in organizations going forward.