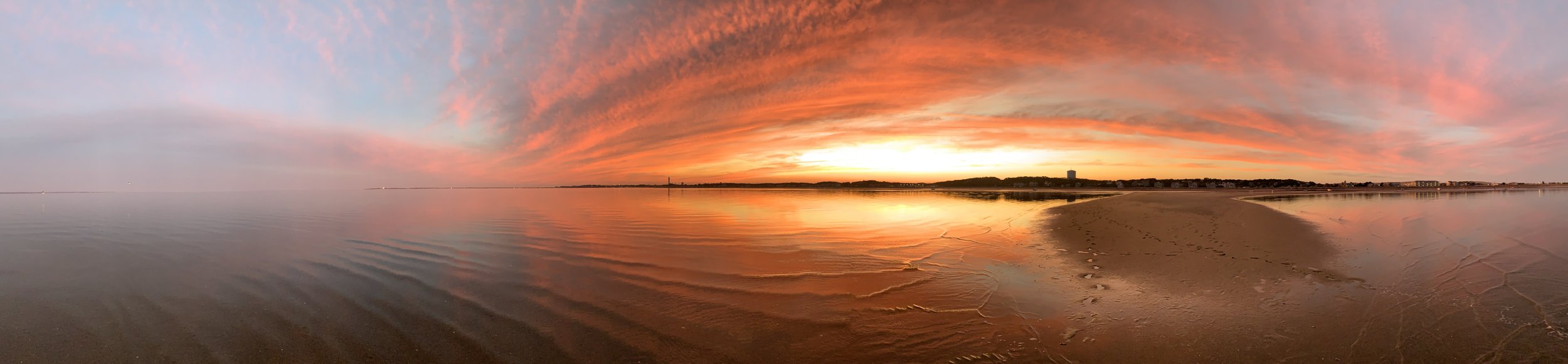

A Moment of Reckoning for Humanitarian and Public Sector
Leaders of these organizations now face a moment of reckoning and—in the chaos of that moment—an opportunity to craft the modern, technologically nimble institutions resilient enough to continue delivering the crucial services upon which their publics so desperately depend. They must use technology to drive out cost, with the core thesis being that to emerge stronger, they must become more modern organizations that use technology to reduce cost and improve services, rather than viewing technology as an ever-expanding cost center. Those that seize the opportunity may survive. Those who let it slip away will fail.

Applying Modern Ecosystem-Oriented Architecture in PubSec
We’ve covered the Principles of Ecosystem-Oriented Architecture (EOA) and Mapping your Cloud Ecosystem in previous articles. We’ll now make the concepts discussed in those previous articles more real in context of Public Sector organizations. To do so, let’s spend some time speaking less about technology and begin describing workloads that incorporate functions and scenarios upon which a typical agency might rely on its cloud ecosystem to perform.
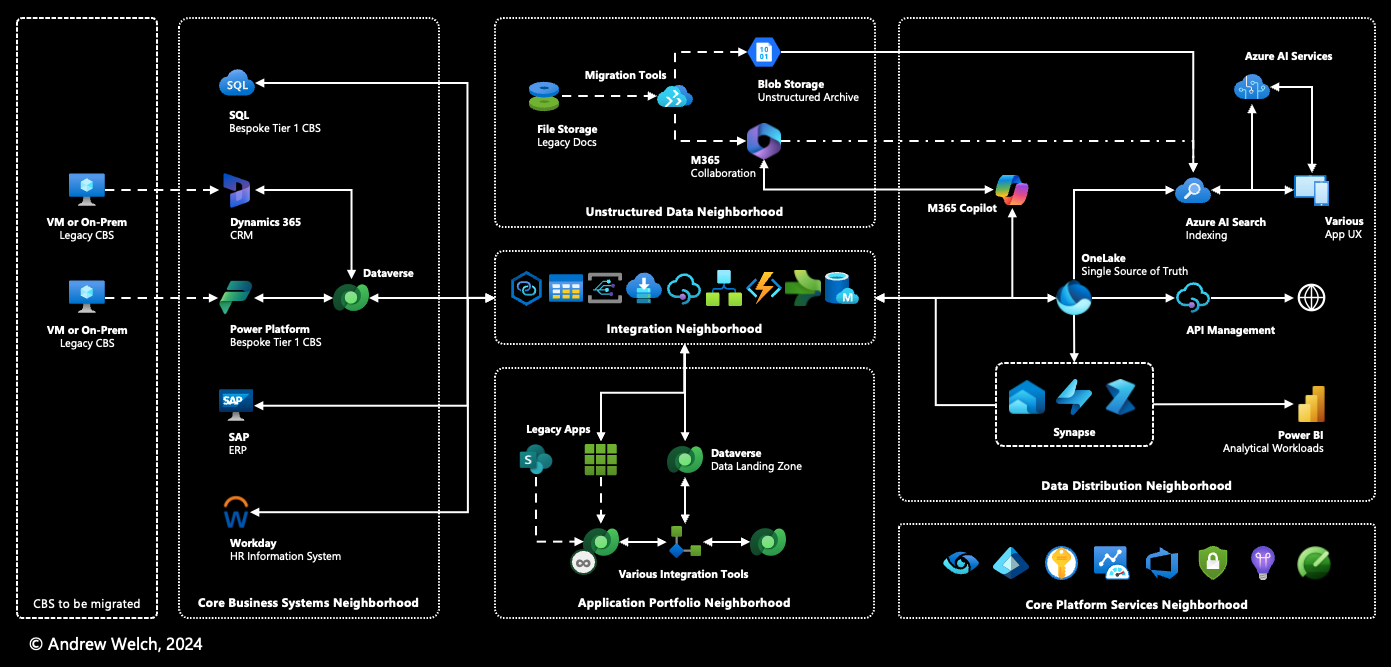
Mapping your Cloud Ecosystem: The Ecosystem Map
"Ecosystem Map" is both one of the 25 dimensions of the AI Strategy Framework and a foundational concept in ecosystem-oriented architecture (EOA), which makes this article doubly important reading for strategic thinkers on both fronts. The “map” metaphor is instructive here. It is used to distinguish an ecosystem map from the various forms of architectural diagrams, nearly all of which tend to include more technical minutiae than a typical ecosystem map. Whereas an architectural diagram provides specific parameters for specific technical solutions, an ecosystem map presents a higher-level, more visionary view of an organization’s cloud ecosystem. This analogy is fundamental to understanding and practicing EOA.

Principles of Ecosystem-Oriented Architecture
We were working with eight to ten years between major disruptions from the dawn of the consumer internet. But these “wave periods”, that is, the time between the crest of two waves, have shortened to three to five years since the rise of the public cloud. It makes sense: As the evolution of computing technology and capacity picks up steam, it similarly accelerates. Innovation begets innovation. Generative AI was only made possible by the incredible computing power and connectivity available in the cloud. Now, AI is further accelerating this pace of change, shortening the time we have available before new waves crash upon the shore. The grace period for organizations to get their act together and position themselves for the next wave is growing much shorter; the margin for error is much narrower.
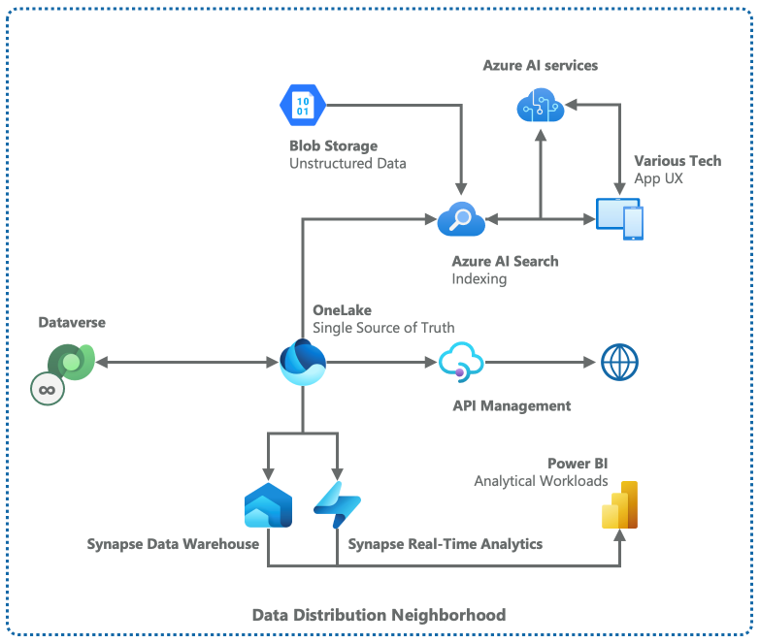
Data Distribution in Power Platform
When taking on the question of how Power Platform integrates with Azure data services, Point-to-Point, Data Consolidation, Master Data Node, and Data Distribution evolve a similar theme. Specifically, each focuses primarily on transactional data during any given users interaction with it. “Data Distribution” is different, focusing more on data distributed for analytics, enterprise search, integration with third-party or external sources via API, data science workloads, or training or augmenting a large language model (LLM). This blog overviews the Data Distribution pattern.
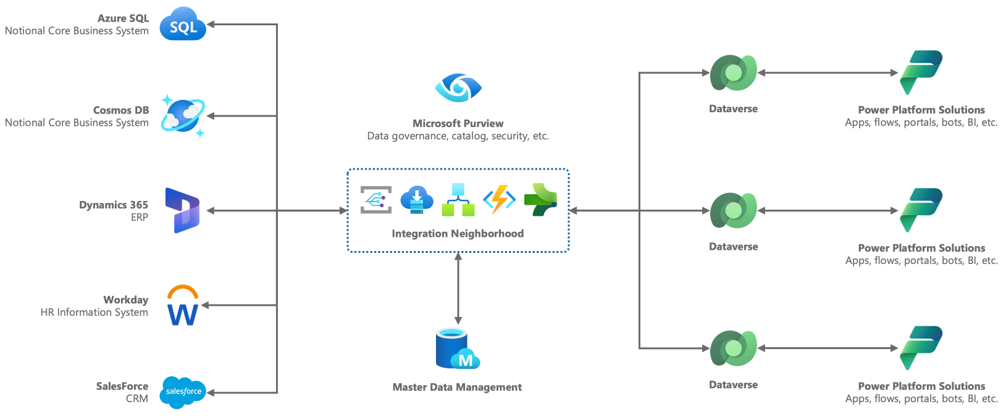
Five strategies to integrate Power Platform in your data platform architecture
When comparing architectural models for Power Platform, it’s important to avoid the instinct to choose just one. Instead, the goal is to explore various approaches that enable different scenarios for integrating Power Platform solutions with enterprise data. Each organization should strategically mix and match these approaches, considering factors like performance, flexibility, maintainability, and cost. This strategy allows for creating adaptable patterns within a cloud ecosystem where Power Platform plays a key role.
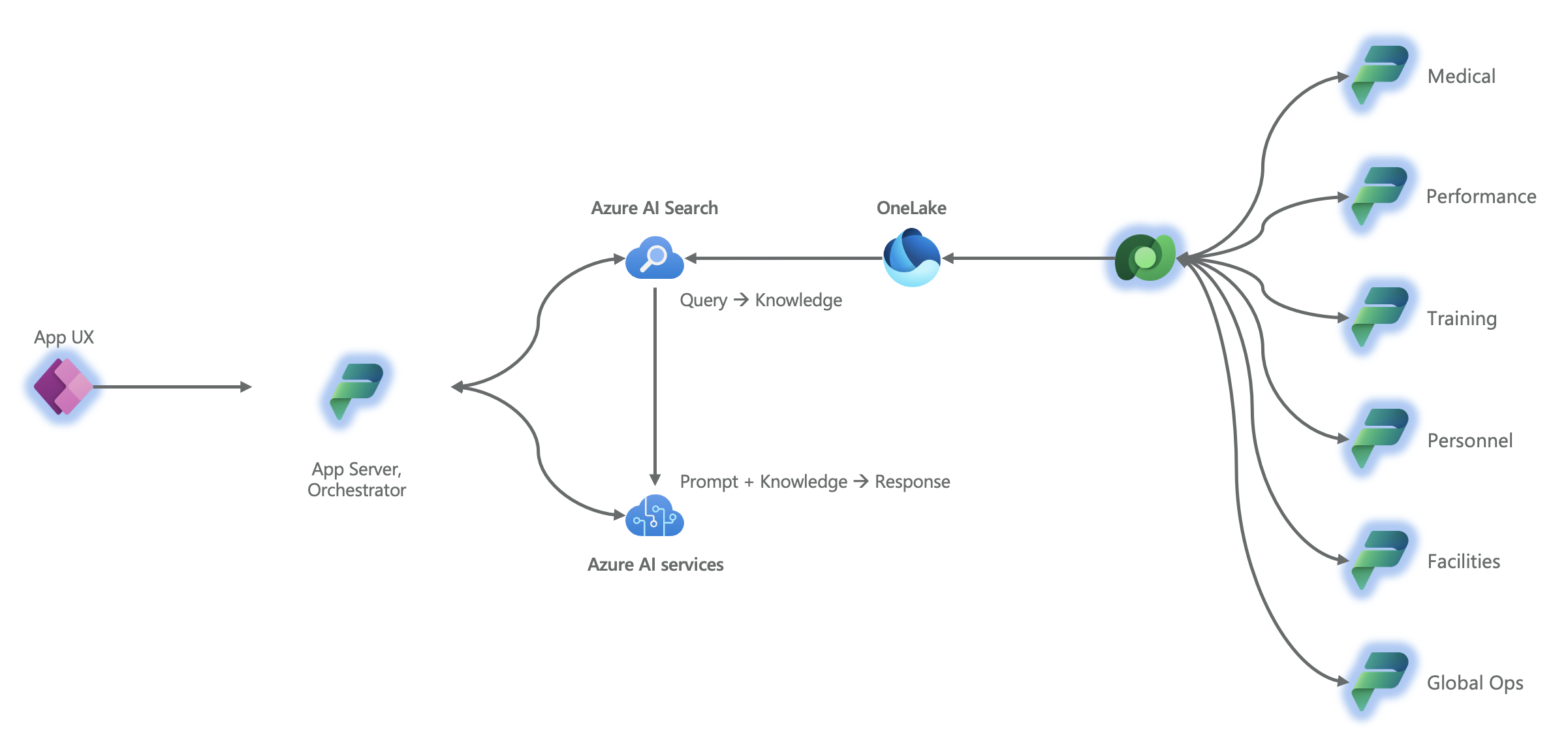
How Power Platform scales generative AI across an organization
Power Platform scales AI and the data platform by providing a composable means of both data collection and delivery of insights and AI capability back to the user. Meanwhile, the great, often unsung capability of Power Platform is not the “app”, rather the ability (via Dataverse) of data transacted in a Power Platform solution to hydrate downstream data distribution scenarios such as analytical workloads, enterprise search, and—you guessed it—whatever AI infused workload you dream up. Let’s explore this.
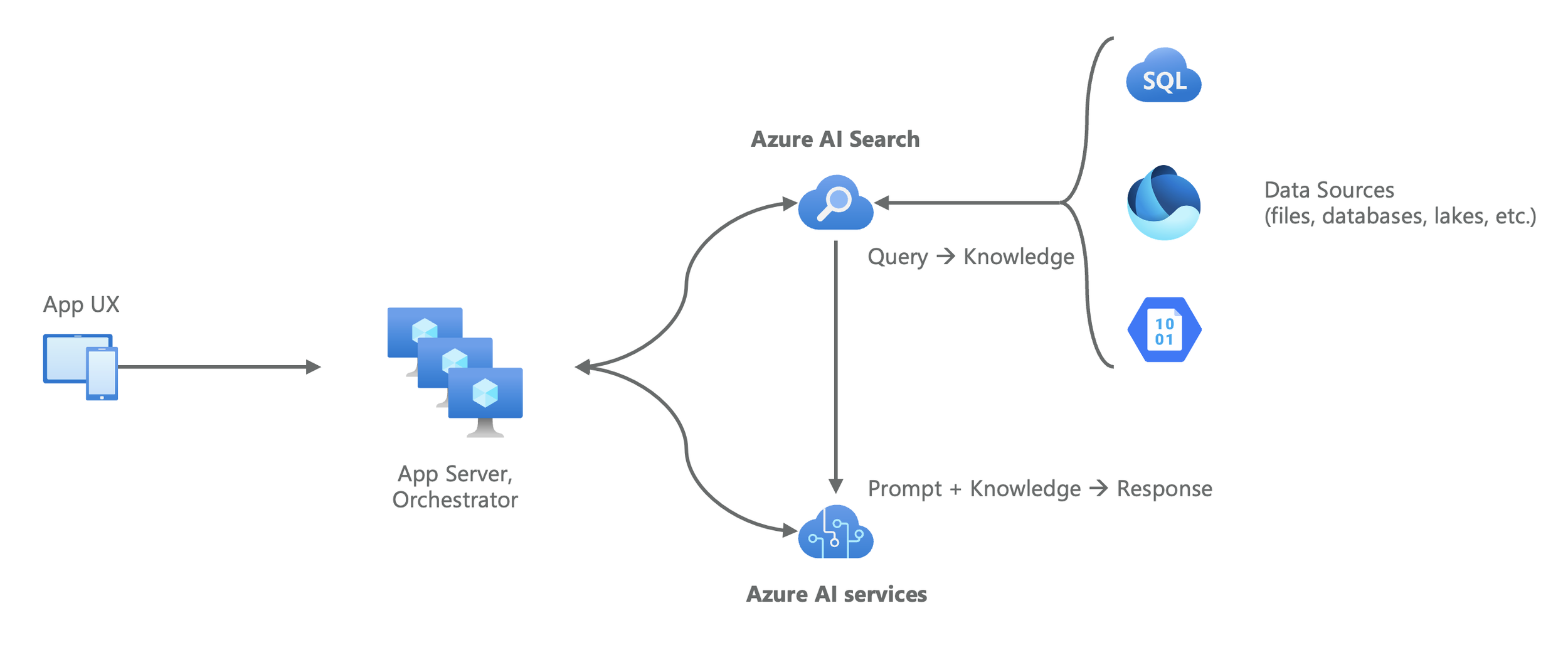
RAG and the fundamentals of AI acting on enterprise data
CIOs and enterprise architects need not be experts in the technical mechanics of AI to formulate and execute an effective AI strategy. That said, it is critical that leaders driving their AI strategy understand this basic concept of how institutional AI—that is to say, AI workloads specific to your organization—both requires and acts on enterprise data. This approach is what we call “Retrieval Augmented Generation” or “RAG”, which you may have heard of. The name is quite literal: Here we are augmenting the generative pre-trained (and now you know what “GPT” stands for) model with data that we have retrieved from the organization’s data estate.
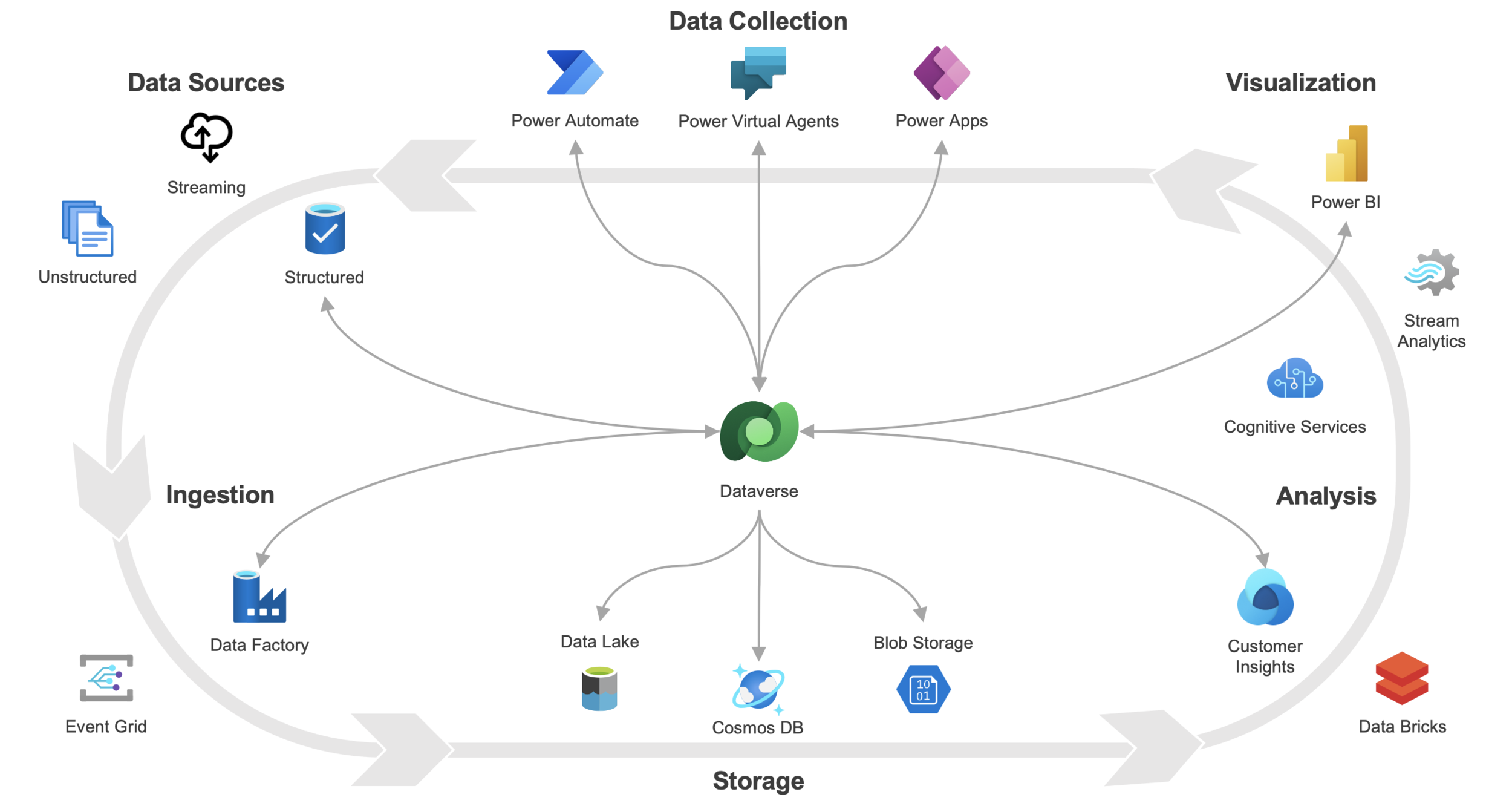
Power Platform in a Modern Data Platform Architecture
I’ve been thinking quite a bit lately about Power Platform as one of the three principal components of the one Microsoft Cloud, alongside Azure and Microsoft 365 of course. This is particularly important in more complex data ecosystem, one of the enterprise management dimensions you’ll find in the Power Platform Adoption Framework. So I want to expand on the “data ecosystem” concept with the idea that modern data platform architecture is a wheel or a cycle (rather than a linear flow), particularly when Power Platform solutions are leveraged (and they should be).